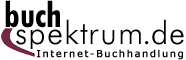 Neuerscheinungen 2012Stand: 2020-01-07 |
Schnellsuche
ISBN/Stichwort/Autor
|
Herderstraße 10 10625 Berlin Tel.: 030 315 714 16 Fax 030 315 714 14 info@buchspektrum.de |
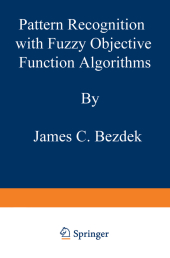
James C. Bezdek
Pattern Recognition with Fuzzy Objective Function Algorithms
2012. 272 S. 229 mm
Verlag/Jahr: SPRINGER, BERLIN 2012
ISBN: 1-475-70452-6 (1475704526)
Neue ISBN: 978-1-475-70452-5 (9781475704525)
Preis und Lieferzeit: Bitte klicken
The fuzzy set was conceived as a result of an attempt to come to grips with the problem of pattern recognition in the context of imprecisely defined categories. In such cases, the belonging of an object to a class is a matter of degree, as is the question of whether or not a group of objects form a cluster. A pioneering application of the theory of fuzzy sets to cluster analysis was made in 1969 by Ruspini. It was not until 1973, however, when the appearance of the work by Dunn and Bezdek on the Fuzzy ISODATA (or fuzzy c-means) algorithms became a landmark in the theory of cluster analysis, that the relevance of the theory of fuzzy sets to cluster analysis and pattern recognition became clearly established. Since then, the theory of fuzzy clustering has developed rapidly and fruitfully, with the author of the present monograph contributing a major share of what we know today. In their seminal work, Bezdek and Dunn have introduced the basic idea of determining the fuzzy clusters by minimizing an appropriately defined functional, and have derived iterative algorithms for computing the membership functions for the clusters in question. The important issue of convergence of such algorithms has become much better understood as a result of recent work which is described in the monograph.
1. Models for Pattern Recognition.- S1 Pattern Recognition.- S2 Some Notes on Mathematical Models.- S3 Uncertainty.- 2. Partitions and Relations.- S4 The Algebra of Fuzzy Sets.- S5 Fuzzy Partition Spaces.- S6 Properties of Fuzzy Partition Spaces.- S7 Fuzzy Relations.- 3. Objective Function Clustering.- S8 Cluster Analysis: An Overview.- S9 Density Functionals.- S10 Likelihood Functionals: Congenital Heart Disease.- S11 Least-Squares Functionals: Fuzzy c-Means.- S12 Descent and Convergence of Fuzzy c-Means.- S13 Feature Selection for Binary Data: Important Medical Symptoms.- 4. Cluster Validity.- S14 Cluster Validity: An Overview.- S15 The Partition Coefficient: Numerical Taxonomy.- S16 Classification Entropy.- S17 The Proportion Exponent: Numerical Taxonomy Revisited.- S18 Normalization and Standardization of Validity Functionals.- S19 The Separation Coefficient: Star-Tracker Systems.- S20 Separation Indices and Fuzzy c-Means.- 5. Modified Objective Function Algorithms.- S21 Affinity Decomposition: An Induced Fuzzy Partitioning Approach.- S22 Shape Descriptions with Fuzzy Covariance Matrices.- S23 The Fuzzy c-Varieties Clustering Algorithms.- S24 Convex Combinations: Fuzzy c-Elliptotypes.- 6. Selected Applications in Classifier Design.- S25 An Overview of Classifier Design: Bayesian Classifiers.- S26 A Heuristic Approach to Parametric Estimation: Bayesian Classifiers for Mixed Normal Distributions.- S27 Nearest Prototype Classifiers: Diagnosis of Stomach Disease.- S28 Fuzzy Classification with Nearest Memberships.- References.- Algorithm Index.- Author Index.