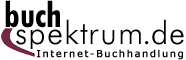 Neuerscheinungen 2015Stand: 2020-02-01 |
Schnellsuche
ISBN/Stichwort/Autor
|
Herderstraße 10 10625 Berlin Tel.: 030 315 714 16 Fax 030 315 714 14 info@buchspektrum.de |
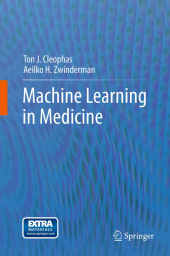
Ton J. M. Cleophas, Aeilko H. Zwinderman
(Beteiligte)
Machine Learning in Medicine
2013. 2015. xv, 265 S. 44 SW-Abb., 80 Tabellen. 235 mm
Verlag/Jahr: SPRINGER NETHERLANDS; SPRINGER 2015
ISBN: 9400793634 (9400793634)
Neue ISBN: 978-9400793637 (9789400793637)
Preis und Lieferzeit: Bitte klicken
This handy guide will help clinicians with computationally intensive methods, like factor analysis, cluster analysis, and discriminant analysis. It includes step by step data analyses in SPSS.
Machine learning is a novel discipline concerned with the analysis of large and multiple variables data. It involves computationally intensive methods, like factor analysis, cluster analysis, and discriminant analysis. It is currently mainly the domain of computer scientists, and is already commonly used in social sciences, marketing research, operational research and applied sciences. It is virtually unused in clinical research. This is probably due to the traditional belief of clinicians in clinical trials where multiple variables are equally balanced by the randomization process and are not further taken into account. In contrast, modern computer data files often involve hundreds of variables like genes and other laboratory values, and computationally intensive methods are required. This book was written as a hand-hold presentation accessible to clinicians, and as a must-read publication for those new to the methods.
Preface.- 1 Introduction to machine learning.- 2 Logistic regression for health profiling.- 3 Optimal scaling: discretization.- 4 Optimal scaling: regularization including ridge, lasso, and elastic net regression.- 5 Partial correlations.- 6 Mixed linear modelling.- 7 Binary partitioning.- 8 Item response modelling.- 9 Time-dependent predictor modelling.- 10 Seasonality assessments.- 11 Non-linear modelling.- 12 Artificial intelligence, multilayer Perceptron modelling.- 13 Artificial intelligence, radial basis function modelling.- 14 Factor analysis.- 15 Hierarchical cluster analysis for unsupervised data.- 16 Partial least squares.- 17 Discriminant analysis for Supervised data.- 18 Canonical regression.- 19 Fuzzy modelling.- 20 Conclusions. Index.
From the reviews:
"This novel book on machine learning in medicine deals with statistical methods for analyzing complex data involving multiple variables. ... The intended audience includes physicians, clinical researchers, physicians in training, statisticians, and medical students, as well as master´s and doctoral students in epidemiology and biostatistics. ... The language is simple and the chapters are well organized. This will be an excellent resource for a quick review of machine learning in medicine, particularly in genetic research, clinical trials, and adverse drug surveillance." (Parthiv Amin, Doody´s Book Reviews, September, 2013)