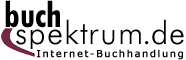 Neuerscheinungen 2016Stand: 2020-02-01 |
Schnellsuche
ISBN/Stichwort/Autor
|
Herderstraße 10 10625 Berlin Tel.: 030 315 714 16 Fax 030 315 714 14 info@buchspektrum.de |
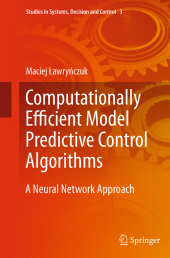
Maciej Lawrynczuk
Computationally Efficient Model Predictive Control Algorithms
A Neural Network Approach
Softcover reprint of the original 1st ed. 2014. 2016. xxiv, 316 S. 87 SW-Abb. 235 mm
Verlag/Jahr: SPRINGER, BERLIN; SPRINGER INTERNATIONAL PUBLISHING 2016
ISBN: 3-319-35021-8 (3319350218)
Neue ISBN: 978-3-319-35021-9 (9783319350219)
Preis und Lieferzeit: Bitte klicken
This book discusses computationally efficient (suboptimal) Model Predictive Control (MPC) techniques based on neural models. Covers feed forward perceptron neural models, neural Hammerstein models and more with high accuracy and computational efficiency.
This book thoroughly discusses computationally efficient (suboptimal) Model Predictive Control (MPC) techniques based on neural models. The subjects treated include:
ú A few types of suboptimal MPC algorithms in which a linear approximation of the model or of the predicted trajectory is successively calculated on-line and used for prediction.
ú Implementation details of the MPC algorithms for feed forward perceptron neural models, neural Hammerstein models, neural Wiener models and state-space neural models.
ú The MPC algorithms based on neural multi-models (inspired by the idea of predictive control).
ú The MPC algorithms with neural approximation with no on-line linearization.
ú The MPC algorithms with guaranteed stability and robustness.
ú Cooperation between the MPC algorithms and set-point optimization.
Thanks to linearization (or neural approximation), the presented suboptimal algorithms do not require demanding on-line nonlinear optimization. The presented simulation results demonstrate high accuracy and computational efficiency of the algorithms. For a few representative nonlinear benchmark processes, such as chemical reactors and a distillation column, for which the classical MPC algorithms based on linear models do not work properly, the trajectories obtained in the suboptimal MPC algorithms are very similar to those given by the ``ideal´´ MPC algorithm with on-line nonlinear optimization repeated at each sampling instant. At the same time, the suboptimal MPC algorithms are significantly less computationally demanding.
MPC Algorithms.-
MPC Algorithms Based on Double-Layer Perceptron
Neural Models: the Prototypes.-
MPC Algorithms Based on Neural Hammerstein and
Wiener Models.-
MPC Algorithms Based on Neural State-Space Models.-
MPC Algorithms Based on Neural Multi-Models.-
MPC Algorithms with Neural Approximation.-
Stability and Robustness of MPC Algorithms.-
Cooperation Between MPC Algorithms and Set-Point
Optimisation Algorithms.
"The book represents a good read for those wishing to study and implement Model Predictive Control (MPC) algorithms based on neural network type models. ... The presentation of the material in the book is pedagogical and includes the ´prototype´ nonlinear MPC problem, which is seen as an ´ideal´ for suboptimal schemes issues from the linearization-based approaches." (Sorin Olaru, Mathematical Reviews, April, 2017)
"This is a monographic work that reflects a large experience in the exploitation of neural network scenarios for Model Predictive Control (MPC). The book provides a rigorous and self-contained material for some key theoretical topics, accompanied by the description of the associated algorithms. ... The exposition is suitable for graduate studies or specialized research stages and requires a medium level of training in control systems engineering." (Octavian Pastravanu, zbMATH 1330.93002, 2016)