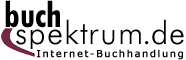 Neuerscheinungen 2010Stand: 2020-01-07 |
Schnellsuche
ISBN/Stichwort/Autor
|
Herderstraße 10 10625 Berlin Tel.: 030 315 714 16 Fax 030 315 714 14 info@buchspektrum.de |
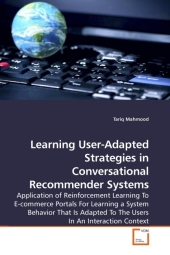
Tariq Mahmood
Learning User-Adapted Strategies in Conversational Recommender Systems
Application of Reinforcement Learning To E-commerce Portals For Learning a System Behavior That Is Adapted To The Users In An Interaction Context
2010. 224 S.
Verlag/Jahr: VDM VERLAG DR. MÜLLER 2010
ISBN: 3-639-07979-5 (3639079795)
Neue ISBN: 978-3-639-07979-1 (9783639079791)
Preis und Lieferzeit: Bitte klicken
This book introduces, describes and validates a novel technology for Conversational Recommender Systems (CRSs). It is targeted for researchers, teachers and students related to the fields of Machine Learning and/or E-commerce. Specifically, CRSs are intelligent E-commerce applications that assist users by supporting an interactive recommendation process. To this end, CRSs employ some type of a recommendation strategy, i.e., a specification of the system behavior. Typically, this strategy is pre-determined in advance and hard-coded inside the system, thus making it possibly non-adapted to the dynamic needs of the users. The technology presented in this book allows CRSs to autonomously learn the optimal (best) strategy for a given recommendation context, from amongst a set of available ones. The optimal strategy is best adapted to the users´ needs, and is learned using Reinforcement Learning techniques (a branch of Machine Learning). We have validated this technology through simulations as well as in an online evaluation involving several hundreds of real users. Our results justify the application of this technology in state-of-the-art E-commerce portals.
Tariq Mahmood is an Assistant Professor at the National University (NUCES), Pakistan. He holds a PhD, with a specialization in the application of Machine Learning techniques to E-commerce systems. His research interests include Machine Learning, Data Mining, Knowledge Discovery in Databases, and the application of Agents to the Semantic Web.