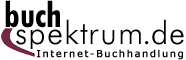 Neuerscheinungen 2011Stand: 2020-01-07 |
Schnellsuche
ISBN/Stichwort/Autor
|
Herderstraße 10 10625 Berlin Tel.: 030 315 714 16 Fax 030 315 714 14 info@buchspektrum.de |
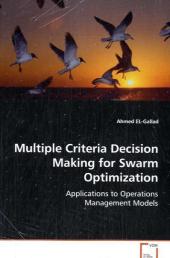
Ahmed El Gallad
Multiple Criteria Decision Making for Swarm Optimization
Applications to Operations Management Models
2011. 200 p. 22 cm
Verlag/Jahr: VDM VERLAG DR. MÜLLER 2011
ISBN: 3-639-06416-X (363906416X)
Neue ISBN: 978-3-639-06416-2 (9783639064162)
Preis und Lieferzeit: Bitte klicken
This book presents a new formulation for the swarm
optimization technique as a system of autonomous
agents. The proposed technique is based on an
intimate understanding of swarms and animal
aggregations in an attempt to simulate cognitive
thinking of their members. The dynamic balance
between gregarious and social intolerance behaviors
demonstrated by social animals is used to form the
swarm and keep its persistence. In this work, members
of the swarm are represented by agents that enjoy a
certain degree of freewill to respond adaptively to
changes on the states of their swarm mates. Adaptive
responses are reflected on the way agents move inside
the problem domain. A new set of basic behaviors is
defined, namely imitation, memory retrieval,
momentum, and play. A multi-criterion decision making
process (MCDM) is employed to update positions of
swarm members in the problem space. Decision making
alternatives are defined from the set of basic
behaviors. Fitness and diversity characterize the
decision criteria that are used to measure the
performance of each alternative. Levenshtien edit
distance is used to measure the distance between
agents in the genotype space. Criteria are then
standardized by means of fuzzy sets. Fuzzified values
of criteria are aggregated by the fuzzy ordered
weighted average (OWA) to reach a single evaluation
function. The overall decision making process is
made to promote both fitness and diversity. The
proposed technique is tested using the traveling
salesmen (TSP) and quadratic assignment problems
(QAP). Results and comparisons show that the
technique outperforms the traditional particle swarm
optimizer (PSO). Also, a comparison of the proposed
technique with the standard genetic algorithm (SGA)
shows that comparable results can be obtained. An
extension of the proposed technique is also proposed
to solve optimization problems with continuous
variables. For this class of optimization, a large
set of diverse benchmark problems is used to test the
proposed technique. A comparison of the performance
with the simple evolutionary algorithm SEA and many
other particle swarm variants is also carried out.
Results show that the proposed technique outperforms
other techniques included in the comparison in almost
all the tested problems.