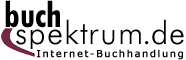 Neuerscheinungen 2011Stand: 2020-01-07 |
Schnellsuche
ISBN/Stichwort/Autor
|
Herderstraße 10 10625 Berlin Tel.: 030 315 714 16 Fax 030 315 714 14 info@buchspektrum.de |
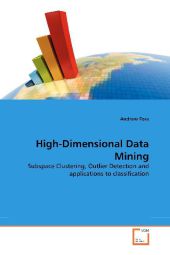
Andrew Foss
High-Dimensional Data Mining
Subspace Clustering, Outlier Detection and applications to classification
2011. 152 S. 220 mm
Verlag/Jahr: VDM VERLAG DR. MÜLLER 2011
ISBN: 3-639-36211-X (363936211X)
Neue ISBN: 978-3-639-36211-4 (9783639362114)
Preis und Lieferzeit: Bitte klicken
Data mining in high dimensionality typically faces the consequences of increasing sparsity and declining differentiation between points, while sparsity tends to increase false negatives. Here, the problem of solving high-dimensional problems using low-dimensional solutions is addressed. In clustering, we provide a new framework for finding candidate subspaces and the clusters within them using only two-dimensional clustering. It is robust to noise and handles overlapping clusters. In the field of outlier detection, several novel algorithms suited to high-dimensional data are presented.m These outperform state-of-the-art outlier detection algorithms in ranking outlierness for many datasets regardless of whether they contain rare classes or not. This approach can be a powerful means of classification for heavily overlapping classes given sufficiently high dimensionality. This is achieved solely due to the differences in variance among the classes. On some difficult datasets, this unsupervised approach yielded better separation than the very best supervised classifiers. This opens a new field in data mining, classification through differences in variance rather than spatial location.
Andrew Foss received his BA in Physics and MA from St John´´s College, Oxford and an MSc and PhD in Computing Science from the University of Alberta. He has worked extensively in IT, developing software that sells internationally. His particular interest is in forcasting.